The Rise of Autonomous AI: Preparing for the Future of Decision-Making
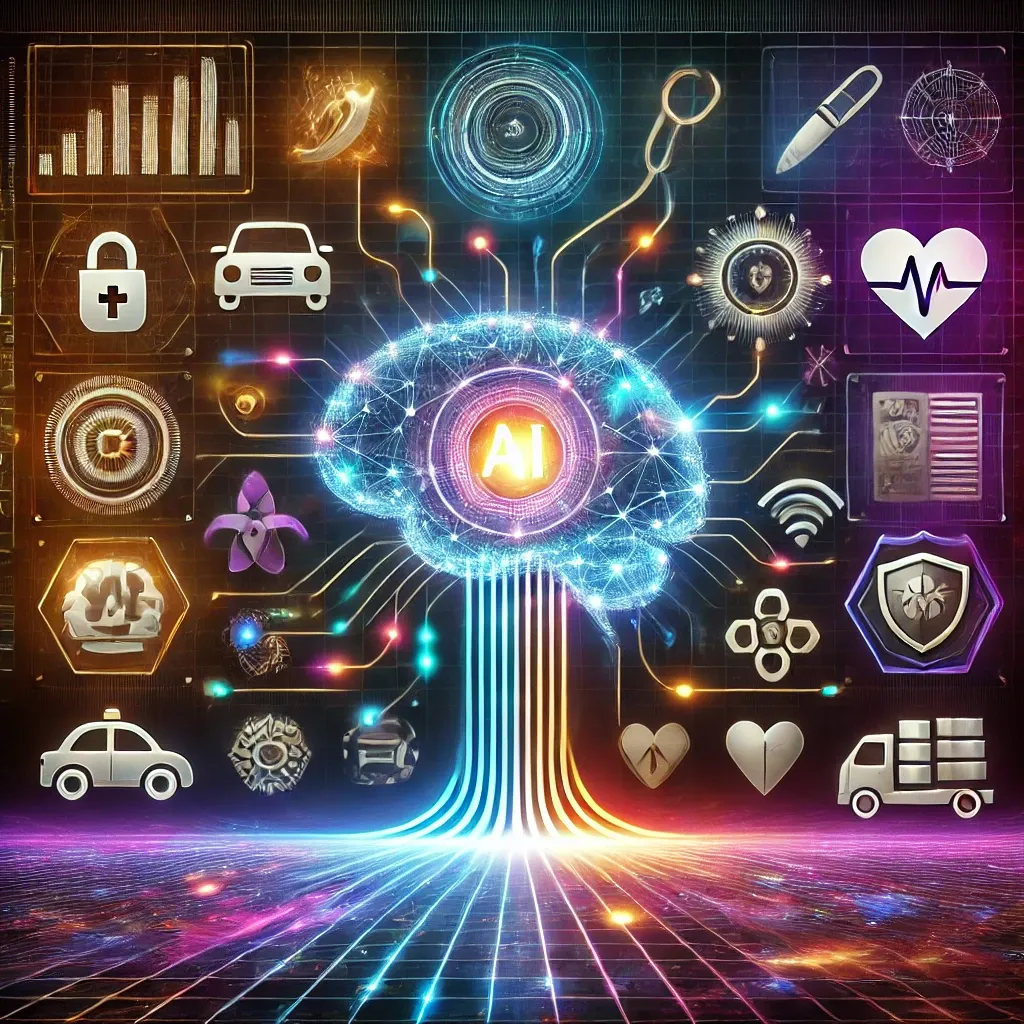
Introduction: The Age of Autonomous AI
Artificial Intelligence (AI) is evolving from a decision-making assistant to an independent decision-maker. Through advanced machine learning, autonomous AI executes tasks, adapts to changing environments, and optimizes processes—all without human intervention.
This transformative capability brings critical challenges, from ethical dilemmas to security risks. Organizations must balance innovation with responsibility to maximize its potential.
What is Autonomous AI?
Autonomous AI systems make decisions using real-time data and predefined goals. Unlike traditional AI that depends on human guidance, autonomous AI continuously learns, adapts, and improves through feedback.
Core Features:
- Self-Learning: Autonomous AI evolves without explicit reprogramming.
- Real-Time Decision-Making: It responds to changing environments and dynamic data.
- Adaptability: It refines strategies based on contextual feedback.
Applications of Autonomous AI
1. Autonomous Vehicles
Self-driving cars use autonomous AI to process sensor data, make instant decisions, and navigate safely.
- Example: AI-powered taxis optimize routes and avoid traffic autonomously.
- Impact: Reduced human error and increased accessibility in transportation.
2. Healthcare
AI systems conduct autonomous diagnostics, assist surgeries, and monitor patients in real time.
- Example: AI tools identify abnormalities in medical imaging for faster diagnosis.
- Impact: Improved accuracy and reduced workload for healthcare professionals.
3. Industrial Automation
Factories use autonomous AI for supply chain management, production optimization, and predictive maintenance.
- Example: Robots adjust to real-time production changes, minimizing downtime.
- Impact: Enhanced productivity and cost savings.
4. Cybersecurity
Autonomous AI detects and counters cyber threats instantly.
- Example: AI quarantines compromised systems during attacks, containing damage.
- Impact: Faster threat response and improved system resilience.
Challenges of Autonomous AI
1. Ethical Concerns
- Accountability: Responsibility for AI decisions remains unclear.
- Bias: AI systems can perpetuate biases from training data.
2. Security Risks
- Adversarial Attacks: Malicious inputs can manipulate AI decisions.
- Data Vulnerabilities: Compromised data affects decision quality.
3. Trust and Transparency
Autonomous AI's "black box" nature makes its decision-making process hard to understand and trust.
Strategies to Prepare for Autonomous AI
1. Implement Explainable AI (XAI)
Create AI systems with transparent decision processes.
- Example: Tools like SHAP and LIME help interpret AI models.
2. Develop Ethical Guidelines
Create frameworks for accountability, fairness, and inclusion.
- Example: IEEE and ISO lead AI ethics standardization efforts.
3. Strengthen Security Measures
Shield AI systems through robust testing and secure data handling.
- Example: Adversarial training builds resistance to attacks.
4. Adopt Continuous Monitoring
Track AI performance in real time to ensure proper function and detect anomalies.
5. Collaborate Across Sectors
Build partnerships among academia, industry, and government to tackle shared challenges.
- Example: The Partnership on AI enables cross-sector collaboration.
Future of Autonomous AI
1. AI-Augmented Decision-Making
Autonomous AI will enhance human decision-making through collaboration.
2. Regulatory Evolution
Governments will create comprehensive regulations for safe, ethical AI deployment.
3. AI in Emerging Fields
Autonomous AI will expand into areas like disaster response and personalized education.
Conclusion: Balancing Innovation and Responsibility
Autonomous AI drives efficiency and innovation across industries. Yet its adoption requires strong ethical standards, transparency, and security measures. Organizations that prepare now will better harness AI's potential while managing its challenges.